The implications of large language models in physical security
AI offers great potential for automation and cost savings. But is AI safe? Explore how artificial intelligence is evolving in our industry today.
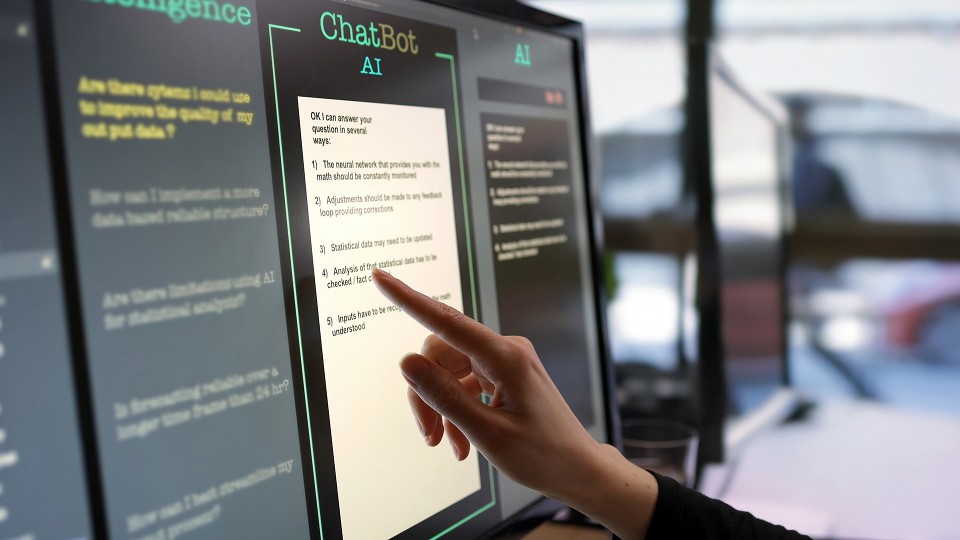
Large language models (LLMs) have recently taken the world by storm. Only months after OpenAI launched its artificial intelligence (AI) chatbot, ChatGPT, it amassed over 100 million users. This makes it the fastest-growing consumer application in history.
And there’s little wonder why. LLMs can do everything from answering questions and explaining complex topics to drafting full-length movie scripts and even writing code. Because of this, people everywhere are both excited and worried about the capabilities of this AI technology.
Although LLMs have recently become a hot topic, it’s worth noting that the technology has been around for a long time. Though with advancements underway, LLMs and other AI tools are creating new opportunities to drive greater automation across various tasks. Having a grounded understanding of AI limitations and potential risks is essential.
Clarifying the terminology
Artificial intelligence, machine learning, deep learning—what are the differences? And how do large language models fit in?
Artificial intelligence (AI)
Artificial intelligence
The concept of simulating human intelligence processes by machines. It refers to tools and processes that enable machines to learn from experience and adjust to new situations without explicit programming. In a nutshell, machine learning and deep learning both fall into the category of artificial intelligence.
Machine learning
Artificial intelligence that can automatically learn with very little human interference.
Natural language processing
The process of using artificial intelligence and machine learning to understand the human language and automatically perform repetitive tasks such as spell check, translations, and summarization.
Deep learning
A subset of machine learning that uses artificial neural networks that mimic the human brain’s learning process.
Generative AI
Enables users to quickly generate content based on a variety of inputs such as text and voice, resulting in a variety of outputs in the form of images, video, and other types of security data.
Large language model (LLM)
Artificial intelligence algorithm that uses deep learning techniques and is fed massive amounts of information from the internet.
What’s the difference between artificial intelligence (AI) and intelligent automation (IA)?
Is AI really intelligent? According to our CEO, Pierre Racz, “AI will remain dependent on human oversight and judgment for decades to come. You have the human supply the creativity, and the intuition, and you have the machine do the heavy lifting.”
This is why intelligent automation can more accurately describe AI that we know today. For clarity, let’s break that down:
Automation is when tasks, whether easy or hard, are done without people needing to do them. Once a process is set up in a program, it can repeat itself whenever needed, always producing the same result.
Traditional automation requires a clear definition from the start. Every aspect, from input to output, must be carefully planned and outlined by a person. Once defined, the automated process can be triggered to operate as intended.
Intelligent automation (IA) allows machines to tackle simple or complex processes, without these processes needing to be explicitly defined. IA typically uses machine learning like generative AI and natural language processing to suggest ways to analyze data or take actions based on existing data and usage patterns. This empowers humans with the right information at the right time and ensures they can focus on core activities instead of data patterns and analysis.
Here’s the short summary of differences:
- Artificial intelligence is a tool. Intelligent automation is about outcomes.
- Where AI is the means, IA is the ends.
- We combine AI with other tools such as automation to achieve an outcome, which is IA.
What are the risks of large language models?
In the past, Sam Altman, CEO of OpenAI admitted to shortcomings around bias which brought ChatGPT security into question. Researchers also found that prompting large language models with identities such as 'a bad person' or even certain historical figures generates a sixfold increase in toxic and harmful responses from the machine learning model.
Are large language models safe? When weighing the risks of LLMs, it’s important to consider this—large language models are trained to satisfy the user as their first priority. LLMs also use an unsupervised AI training method to feed off a large pool of random data from the internet. This means the answers they give aren’t always accurate, truthful, or bias-free. All of this becomes extremely dangerous in a security context.
In fact, this unsupervised AI method has opened the door to what’s now coined ‘truth hallucinations’. This happens when an AI model generates answers that seem plausible but aren’t factual or based on real-world data.
Using LLMs can also create serious privacy and confidentiality risks. The model can learn from data that contains confidential information about people and companies. And since every text prompt is used to train the next version, this means someone prompting the LLM about similar content might then become privy to that sensitive information through AI chatbot responses.
Then, there are the more ill-intended abuses of this AI technology. Consider how malicious individuals with little or no programming knowledge could ask an AI chatbot to write a script that exploits a known vulnerability or request a list of ways to hack specific applications or protocols. Though these are hypothetical examples, you can’t help but wonder how these technologies could be exploited in ways we can’t anticipate yet.
How are large language models evolving in the physical security space?
Now that large language models are creating a lot of hype, there’s this sense that AI can magically make anything possible. But not all AI models are the same or advancing at the same pace.
Large language, deep learning, and machine learning models are delivering outcomes based on probability. If we take a closer look at large language models specifically, their main goal is to provide the most plausible answer from a massive and indiscriminate volume of data. As mentioned earlier, this can lead to misinformation or AI hallucinations.
In the physical security space, machine learning and deep learning algorithms don't rely on generative AI, which can invent information. Instead, AI models are built to detect patterns and classify data. Since these outcomes are still based on probability, we need humans to stay in the loop and make the final call on what’s true and not.
That said, the same is true for all the beneficial outcomes of LLMs too. For security applications, there could be a future where operators can use an AI language model within a security platform to get quick answers, such as asking ‘how many people are on the 3rd floor right now?’ or ‘how many visitor badges did we issue last month?’ It can also be used to help organizations create security policies or improve details in response protocols.
No matter how AI language models are used, one thing is certain—security use cases would require approaches where the models run in a more contained environment. So, while there’s a lot of excitement around LLMs right now, a lot of work still needs to be done for them to become safe and feasible for physical security applications.
How is AI being implemented in the physical security space?
LLMs may be top of mind right now, but using AI in physical security is not new. There are many interesting ways that AI is used to support various applications.
Here are some examples of how AI is used in physical security today:
Speeding up investigations
Scouring video of a specific time period to find all footage with a red vehicle.
Automating people counting
Getting alerted to maximum occupancy thresholds in a building or knowing when customer lines are getting too long to enhance service.
Detecting vehicle license plates
Tracking wanted vehicles, streamlining employee parking at offices, or monitoring traffic flow across cities.
Enhancing cybersecurity
Strengthening anti-virus protection on appliances by using machine learning to identify and block known and unknown malware from running on endpoints.
SECURITY CENTER SAAS
Embracing intelligent automation
For most organizations, implementing AI comes down to a couple of driving factors; achieving large-scale data analyses and higher levels of automation. In an era where everyone is talking about digital transformation, organizations want to leverage their physical security investments and data to ramp up productivity, improve operations, and reduce costs.
Automation can also help organizations adhere to various industry standards and regulations while lowering the cost of compliance. That’s because deep learning and machine learning offer the potential to automate a lot of data processing and workflows while pointing operators to relevant insights. This allows them to quickly respond to business disruptions and make better decisions.
For instance, AI is becoming more democratized through various video analytics solutions. Retailers today can use directional flow or crosslines detection analytics to track shoppers’ behavior. Sports stadiums can automatically identify bottlenecks to ease foot traffic during intermissions. Organizations can also use people counting analytics to track occupancy levels to meet evolving safety regulations.
Is it too good to be true?
Even with these out-of-box solutions at hand, there are still a lot of myths out there about what AI can and cannot do. So, it’s important for professionals to understand that most AI solutions in physical security aren’t one-size-fits-all. This is especially true for those who are looking to leverage AI techniques to solve specific business problems.
Automating tasks or reaching a desired outcome is a process of determining technical feasibility. It involves identifying the existing solutions that are in place, other technologies that might be needed, and whether there will be compatibility issues to work out or other environmental factors to consider.
Even when feasibility is assessed, some organizations may wonder whether the investment justifies the outcome. So, while AI is a key component to reaching higher levels of automation in the physical security industry, there is still much consideration, forethought, and planning required to achieve accurate results.
In other words, it’s crucial to be cautious when exploring new AI solutions, and their promised outcomes need to be weighed with critical thinking and due diligence.
What are the best ways to capitalize on AI in physical security today?
AI-enabled applications are advancing in new and exciting ways. They show great promise in helping organizations achieve specific outcomes that heighten productivity, security, and safety across their organization.
One of the best ways to capitalize on new AI advances in physical security is by implementing an open security platform. Open architecture gives security professionals the freedom to explore artificial intelligence applications that drive greater value across their operations. As AI solutions come to market, leaders can try out these applications, often for free, and select the ones that best fit their objectives and environment.
As new opportunities emerge, so do new risks. That’s why it’s equally important to partner with organizations that prioritize data protection, privacy, and the responsible use of AI. This will not only help enhance cyber resilience and foster greater trust in your organization, but it’s also part of being socially responsible.
In the IBM report AI Ethics in Action, 85% of consumers said that it is important for organizations to factor in ethics as they use AI to tackle society’s problems. 75% of executives believe getting AI ethics right can set a company apart from its competitors. And more than 60% of executives see AI ethics helping their organizations perform better in matters of diversity, inclusion, social responsibility, and sustainability.
Another recent study by Amazon Web Services (AWS) and Morning Consult revealed that 77% of the respondents acknowledge the importance of Responsible AI. While 92% of businesses said they plan to use AI-powered solutions by 2028, almost (47%) said they plan to increase their investment in Responsible AI in 2024. Over one-third (35%) believe that irresponsible AI use could cost their company at least $1 million or otherwise endanger their business.
How Genetec considers privacy and data governance using Responsible AI
Since artificial intelligence algorithms can process large amounts of data quickly and accurately, AI is becoming an increasingly important tool for physical security solutions. But as AI evolves, it magnifies the ability to use personal information in ways that can intrude on privacy.
AI models can also inadvertently produce skewed decisions or results based on various biases. This can impact decisions and ultimately lead to discrimination. While AI has the power to revolutionize how work gets done and how decisions get made, it needs to be deployed responsibly. After all, trust is essential to the continued growth and sustainability of our digital world. And the best way to build trust? Partnering with organizations that prioritize data protection, privacy, and the responsible use of AI.
This is why our team at Genetec™ take Responsible AI seriously. In fact, we’ve devised a set of guiding principles for creating, improving, and maintaining our AI models. These cover the following three core pillars:
![]() |
Privacy and data governance |
As a technology provider, we take responsibility for how we use AI in the development of our solutions. That means we only use datasets that respect relevant data protection regulations. And wherever possible, we anonymize datasets and ethically source, and securely store data used for training machine learning models. We also treat datasets with the utmost care and keep data protection and privacy top of mind in everything we do. This includes sticking to strict authorization and authentication measures to ensure the wrong people don’t get access to sensitive data and information across our AI-driven applications. We’re committed to providing our customers with built-in tools that will help them comply with evolving AI regulations.
![]() |
Trustworthiness and safety |
As we develop and use AI models, we’re always thinking about how we can minimize bias. Our goal is to make sure that our solutions always deliver results that are balanced and equitable. Part of ensuring this means we rigorously test our AI models before we share them with our customers. We also work hard to continuously improve the accuracy and confidence of our models. Finally, we strive to make our AI models explainable. This means that when our AI algorithms decide or deliver an outcome, we’ll be able to tell our customers exactly how it reached that conclusion.
![]() |
Humans in the loop |
At Genetec, we make sure that our AI models can’t make critical decisions on their own. We believe that a human should always be in the loop and have the final say. Because in a physical security context, prioritizing human-centric decision-making is critical. Think of life-or-death situations where humans innately understand the dangers at play and the actions needed to save a life. Machines simply cannot grasp the intricacies of real-life events like a security operator, so relying solely on statistical models cannot be the answer. This is also why we always aim to empower people through augmentation. That means our systems should always drive insights to enhance human capacity for judgment.
